Written in collaboration with Asma Zgolli (this article also appears on Asma’s Medium Page), and Balavivek Sivanantham
At the Rework Enterprise AI Summit in Berlin, Elizabeth Press, Owner and Community Builder of D3M Labs, moderated a panel discussion, „What is the future of AI Adoption?“ together with Balavivek Sivanantham, Technical Lead/Machine Learning Engineer at Bayer and Asma Zgolli, Machine Learning Engineer at Centa MG.
What are some relevant trends?
Many practitioners do not use “AI” as a phrase, instead use the application, namely machine learning and deep learning. Those are the two main applications that have seen exponential growth in research and industry within the last decade.
Graph databases are making new use cases possible, for example in the energy, telecom, finance and healthcare sectors. TigerGraph is one platform for analytics on graph data that offers many tools to get insights from connected data like deep link analysis, as well as clustering and classification techniques.
Low code and no code tools are entering AI, along with other places in the stack. Vertex AI is an example of a low code and no code tool that lowers the coding barrier to entry for people wanting to manipulate data in ways that previously would have required intermediate or advanced python knowledge.
Data lakes avail easier access to data for data scientists and thus have enabled more use cases and applications that were not previously possible. Many vendors who offer data lake solutions. Here is Databricks’ explanation of what a data lake is.
Building a bridge between business and people who develop AI has been an obstacle to putting AI use cases into production. We are still talking about many of the same use cases we were discussing a decade ago.
What is being done to remove barriers between the creators and consumers of AI?
Usability. For example, recommender systems for e-commerce in fashion, data scientists use feedback about the recommended sizes to adjust the usability of the model. The model is updated frequently when new data is collected from the client feedback using techniques like reinforcement learning or online machine learning. In this context, the feedback is collected from post purchase surveys or mined from comments (using NLP algorithms)
Explainable AI. AI is often a backbox. Developers and end customers often don’t understand what is happening with their data. Data scientists need to make a greater effort to explain what they are doing with the data, including being open about the models being used, pros and cons, etc.
Fairness and Robustness. It is important to reassure the consumers that the developers of AI will not influence the outcome of the model and that the model is not hackable. Practices of anonymization of sensitive data and establishing a security and privacy strategy help us enforce these ethics
Not all companies and use cases are created equal. A global healthcare provider such as Bayer has different privacy standards than EdTech platforms, for example.
What are some enablers of privacy, security, and ethical AI?
Privacy has gained increasing importance largely due to GDPR regulation in Europe, but also because of increasing consumer awareness. There are different types of anonymization and processes companies can put in place to make sure personal data is only used as needed and as allowed.
Deployment is often in Edge computing and on premise for sensitive use cases.
Focus on data quality, metadata management, data governance and data catalogs. Not only do these topics make life easier for creators of AI, but make privacy and security easier to manage.
What are some enablers of deploying AI solutions across borders?
Cloud solutions that enable the end-user. Cloud solutions such as Vertex AI enable students, freelancers and other practitioners who do not have access to supercomputers to have access to automatic machine learning models. Some of these cloud solutions can also create models on their own.
MLops, Machine Learning operations. Platforms such as Mlflow manage the life cycle of machine learning models. For example, teams can put the model into production, track and monitor the models.
Multinationals with large amounts of models need many tools. Multi Cloud environments, which all cost money and necessitate management overhead, are often needed for global deployments of multinational companies.
Most models are segmented by a region or a country, not globally.
MLOps. Many Startups work in MLOps level 0 some Level 1, maybe Level 2. Maybe in enterprise area level 2 might be easier? Here is Google’s explanation of MLOps and the different levels.
What is the reality of MLOps in companies, both startup and enterprise?
In startups, there might be one person working on a model, so many startups are at MLOps level 0. No automation and little to no processes.
In a larger organization, one person might work on a niche product, but often there are more nuanced and specialized roles for different steps of the MLOps process and enterprise companies can get up to Level 2. Databricks is a tool many companies use to orchestrate their MLOps on various cloud platforms.
Last decade was good. We are now living in challenging times.
What are opportunities for AI? Is AI an opportunity for us as human beings?
Here are some use cases:
● Smart grids. In an energy crisis, predicting energy costs for private households and businesses is important.
● Predictive maintenance and fraud detection.
● Improved recommender systems due to increased traffic in the Covid crisis.
● Customer support application & conversational AI.
● AI-enhanced drug analysis that enables early stage detection beyond the human eye.
AI collaborating with human intelligence to get a better solution enables us to put less effort into whatever we want to do to get the result we want. It is important to remember that humans create AI. If humans want to do good, then AI will be used for good – or bad.
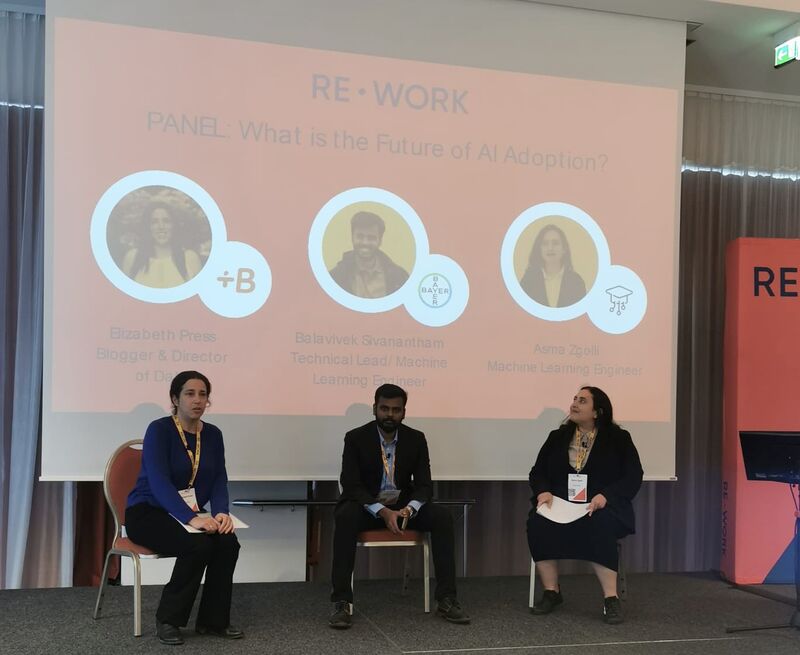
Panel Discussion: What is the future of AI adoption, Rework Enterprise AI Summit in Berlin
Elizabeth Press is a data leader, avid blogger and published author with a track record of building high-performing data organizations and creating strategic advantages out of data.
She has architected robust and impactful organizations, run analytics and strategy projects, as well as provided insights for blue chips and top investors on every continent. A globally minded leader and entrepreneur who has lived and professionally worked in 6 countries across 3 continents, she can manage diversity and inspire cross-functionally – and virtually.
Balavivek Sivanantham is a Data Engineer who loves to play and work with Data. He recently developed a data science tool using Audi Assembly line data that let workers in the assembly line to produce cars with few to zero errors. He’d love to combine his passion for data and machine learning with his data engineering skills to continue building personalized tools to help people and businesses by reducing the cost with predictive analysis.
Asma Zgolli is a Doctor and Engineer in Computer Science with a specialization in Big Data and Applied Machine Learning. Her goal is to be able to make use of her data science and analytics skills to solve real-world problems.