Beyond artisanal data science, how can we scale AI for industry and commercialize AI-innovations? I, Elizabeth Press , sat down with RE•WORK to talk about how we can improve the scalability and industrialization of AI.
Listen to the podcast on Spotify and Anchor FM. Continue reading below for some insights from the podcast.
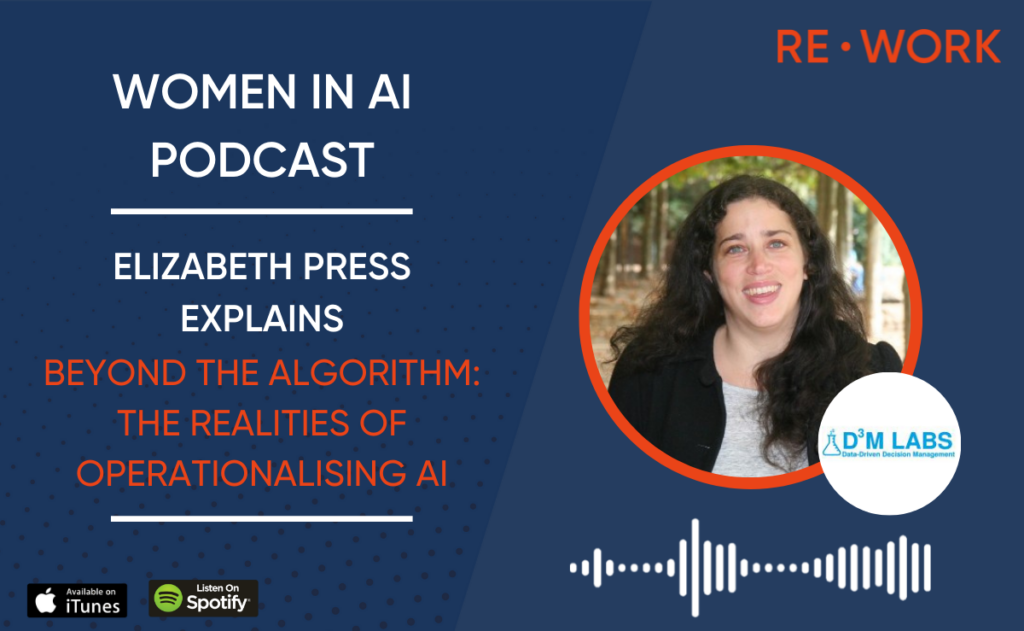
Human-centricity is crucial, even for machine learning:
Customer centricity and the principles of design thinking: Understanding the problem space, the context of the customer, the problem the customer is trying to solve and the environment that the system is being used in is an important step that is often skipped. Observing the environment of the customer will probably give you more hypotheses about the why, which will lead to understanding noise between the signals and result in less confounding variables.
Usability, user experience (UX) as part of the design: An AI solution is usually not just an algorithm, but instead is embedded in an end product, usually a screen-based interface in the form of, for example, a recommendation system to somebody who is probably not a data scientist.
Human centricity is also important for in-house analytics:
Intuitive dashboard design and findability of the dashboard were key factors to stakeholders understanding and using the models my teams developed. Data science and data analysts I managed productionized multitouch attribution and churn predictions models in the form of dashboard visualizations and decision support to stakeholders. Stakeholders should be able to look at dashboards, come to conclusions and decide on next steps easily. Spending time on that last mile of delivery was always well invested.
Making stakeholders do math in their heads because of too many nonvisualized or poorly visualized numbers is one common mistake that creators of internal data products make.
Clicking around to find dashboards is frustrating. Stakeholders do not want to feel frustrated and exhausted every time they want to see a dashboard. Especially, if you don’t want excessive Slacks asking for information that is in dashboards, make the dashboards findable.
MLOps (Machine Leaning Operations) instead of artisanal AI
The error of artisanal AI should come to an end, at least when we talk about industrializing AI solutions. MLOps should be a cornerstone of any company’s AI strategy. Companies, both multinational and startup, agree. Artsy, Google, Shopify, BSH Bosch talked about MLOps at the Rework Enterprise AI Summit in Berlin. Google has a guide to how they define MLOps and different levels of maturity.
MLOps has come into data science from DevOps. An industrial AI competency needs the governance and control mechanisms that DevOps has brought to the software world. One of the main additions MLOps has compared to DevOps is the element of model and schema validation.
MLOps at different levels of company and data maturity
There are different levels of MLOps, as explained in Google’s MLOps guide. It is fair enough that the first data scientist in an early stage startup might need to work in an MLOps Level 0, as an artisanal data scientist. However, if a startup wants to use AI to create a competitive advantage, they should adopt MLOps pretty quickly and work themselves up to MLOps level 1, which includes a testing and production environment, validations of models and schemas and partial automation. Increasing validation and automation mechanisms will enable quality management of algorithmic products.
Data quality is often the biggest hurdle
If the general public could see the data pipelines of many companies, there would be less fear of robots taking over many human tasks. Jumping to data science without getting data engineering in order was putting the cart before the horse on an industrial scale. Getting the data to a quality that is required for an algorithm to be automated, productionized and even industrialized is a huge amount of work. More companies than the data practitioner community would like to admit are far below that level of data quality.
More non-sexy stuff that blocks the industrialization of AI
Scaling across data privacy laws, across datasets in different countries is still something that even multinationals struggle with. The reality under the hood often looks like patchy multi-cloud environments, not understanding regulations, poor operations and tripping over unobservable data and resulting bad data quality.
What is holding back AI in the Berlin tech scene?
Artisanal AI, rather than treating AI like a product that needs to be validated, quality controlled and scaled. For years, artisanal brilliant data scientists were looked upon as a panacea for digitalization. Many founders, intrapreneurs and investors put their hopes that data scientists could be the solutions for the future digital economy. The discussions in the data and tech community focused on code rather than the more usability, operational and commercial aspects.
Reactive investment in AI
Germany is a reactive market to a large extent, following first movers such as the USA and Israel. Were there German startups in AI and/or using AI that German investors put their money in? Yes. Mostly if the concept was proven elsewhere, namely in the USA or Israel.
Risk adversity
Historically local investors in Berlin have had the strategy of investing in business innovation using technologies that have been proven to be commercially viable elsewhere. Often the investments were made on business innovations that have also been proven in other markets.
Over reliance on artisanal AI – not getting the execution right
There was often a view that the data scientist could code and make things happen, from data engineering to sometimes commercialization. Many AI implementations I saw lacked focus on processes or combining development with customer focus or business and sales strategy.
How can we help data scientists be more successful in their craft?
Let them focus on their core competencies. Many data scientists like to focus on the technical aspects of developing algorithms so give them space to do that.
Enable collaboration with data engineers, data product managers and product owners, data analysts, designers and more. Maybe one day, the data scientists would like to move into management or try other roles. Let them do that to!
At an early stage, yes a data scientist might have to do everything, but as the company grows and the AI competency needs to scale, people need to have professional level skillsets for their given tasks.
Three things to remember:
Understand the job that needs to get done.
Understand the requirements skills tools tech
Do not forget the operations
Related links
Why the public needs to know more about AI – An interview with Varsh Anilkumar (who was also at the Enterprise AI Summit)
Thought leadership folloing the Rework Enterprise AI Summit in Berlin