How did you get interested in the economics of data?
After finishing a Bachelor’s degree in Industrial Technologies with a focus on Machine Learning, I went to Munich in 2018 to do my Master’s in Computational Science and Engineering at the Technical University of Munich. My goal at that time was to work as a Deep Learning Engineer. Initially, I only had a technical perspective. My horizons broadened as I got an Honors Degree in Technology Management at CDTM and built a couple of mock startups as part of the program. Through building the mock startups, I came into contact with investors and entrepreneurs. At this point, I started to see that company success was not just about technology, but also profitable growth and controlling operating costs.
How did you develop the four data moat framework?
Whenever I get the opportunity, I read articles and piece them together to form my own perspective.
In 2021 Viet Lee, principal at La Famiglia, released an article about the Data Flywheel. This framework set the foundation of a new perspective for me on evaluating the positioning of data companies. I used this new perspective whenever anybody told me about data startups and new data products.
What is a data moat?
Data moats are valuable attributes of your business that are hard to replicate.
By valuable, I mean that they should be based on your customer’s need or a pain point.
What are the overarching categories of data moats?
There’s two main ways that data strategies can set you apart:
- Insight: Analytics can support decision-makers with the necessary context to steer their businesses towards better outcomes.
- Data-driven product capabilities: Providing your product with new functionalities or dramatically building upon your existing ones by training algorithms with data.
My focus has been on data-driven product capabilities.
What are the types of moats created by data-driven product capabilities?
I distinguish four potential data moats that make your business more defensible in different time horizons. Before we jump into them, please keep in mind that they’re not mutually exclusive, and you may find parallels with moats that apply beyond the data domain.
The four data moats are:
- The Data Flywheel: This moat entails collecting product usage data to drive new capabilities that increase the value of the services delivered to the users. This increases users. More users means more data. The cycle can go on indefinitely.
- Proprietary Data: This moat entails securing exclusive data sources beyond usage data, making it more difficult for competitors to replicate the same use cases.
- Data Aggregation: This moat entails aggregating data to reach a critical quantity or enriching the data so that models can successfully train on it.
- Data Talent: Employer branding is often functional-specific. Building a reputation as a data excellence center will guarantee a talent advantage for the years to come.
What are some barriers that help deepen your moat?
Some moats can exist on day one, while others require a higher level of commercial and technical expertise, tooling, and volume of data.
A certain level of data volume is required to get a data flywheel going. Of course, starting with a large enough dataset gives you a better competitive advantage. Typical examples of companies using a flywheel are Instagram and TikTok.
Deviation from off-the shelf models: Even though off-the-shelf models don’t exist for every use case, you need to refine the model via the data flywheel to gain a competitive advantage.
Proprietary data is something you can collect from day one.
The talent flywheel can start turning by investing in a couple of rockstar hires early on.
Expertise in working with highly confidential data, because not everybody knows how to create and put privacy-preserving models into production. Merk is one example of a company using federated learning for drug discovery.
What are some obstacles to building moats?
Open-sourcing and thought leadership are double-edged swords.
These strategies give visibility to the insides of the company—both the expertise and the lack of it.
What happens in the absence of moats?
Companies can still be perfectly defensible without having data at the core of their business. However, they will have to double down on alternative sources of competitive advantage.
What happens if a company does not have a competitive advantage?
Companies that fail to build a competitive advantage may see their profits squeezed away by competitors that can give the same value to the customers at a lower operating cost.
What role does internal analytics play in building competitive advantages?
Using analytics to enable decisions is foundational to the operations of any business today.
An overview of the critical business metrics allows you to steer your business with unprecedented precision.
It’s not that we should automate away decision-making. Data supports us with a better context in calling the right shots.
What happens when data moats are implemented badly?
Implementing data moats badly can be even more harmful than not having them.
Failing to realize the shortcomings of your strategy will burn your resources and prevent you from focusing on more impactful initiatives.
What does a bad implementation of a data moat look like?
Let’s cover an antipattern for each of the data moats.
- Data flywheel on a product with zero value: Starting with a product that drives zero value to the customer. Even if your product adds some intrinsic value, if you get the flywheel going and capture the wrong data, because you have no strategy of what data to store and have not thought about what data is important. You train your models on unimportant data. You wasted the data collection budget for months without creating any upside value out of data. The forever flywheel of value-free data becomes a bottomless pit in your budget. You will burn through infrastructure spend without adding any value to your business.
- Proprietary data is not used. You only use publicly available data: Only training on publicly available datasets or taking public, pre-trained models without customizing them further will create no deviation that results in competitive differentiation. If you do that, in 2 days, your competitors will have the same precise solutions as you. Then see your competitors run away with your customers.
- Data Aggregation: Carelessly moving customer personal identifiable information (PII) across machines and regions. Life’s too short to think about privacy! Unfortunately, your customers and regulatory authorities disagree.
- Data talent: Being cheap and underpaying the first batch of poor devils who join your company. If someone turns out to be any good, hide them from the world! You don’t want them to be poached by an empowering and fair employer.
What are some first steps to understanding which strategy you want to choose and implementing it?
Assuming that you have done the thorough work of defining your ideal customer profile, user personas, understanding their biggest pains and daily activities, and you understand the positioning of your product.
The steps are as follows:
- Understand the unique value proposition of your product: What job do you promise your ideal customer to do for them or help them with?
- Ask yourself how data can enrich the product: Could we deliver a fundamentally better service by enriching your product with prediction, recommendation, bots, or synthesis capabilities?
If you could come up with solid, sensible answers to both questions, is time to think about choosing the correct moat. Here are some points to help you decide.
- We’re talking about a data flywheel if event-driven product usage data is required to power the enhanced capabilities.
- We’re talking about proprietary data collection if data beyond event-driven product usage data is required. i.e. Inputs into credit scoring, the collection of satellite imagery or any kind of domain-specific data not generated through product usage..
- Aggregation is relevant if each user sits on small subsets of data that are not enough to power the models or if data required for a use case spans multiple systems.
Can you mention a key factor for a successful implementation?
Implementation starts with putting some numbers to the costs of data collection, infrastructure and building data capabilities.
As with any other functionalities, it’s not only about the potential user upside, but about its feasibility, and the cost of building it and maintenance.
Has your data moat strategy evolved over time?
Initially I thought that building a data flywheel would make your company invincible. However, I later realized that data moats need maintenance and development.
Three main learnings:
- Being aware of tools one has to create venture defensibility through data capabilities
- Defensibility strategies evolve over time. After some time your data moats will erode, so it’s important to stack moats that cover for defensibility in the different time horizons..
- Accuracy is not rewarded equally in all verticals and use cases. Where does this use case sit? Is it in the long-tail and thus pushing for more accuracy would be worth it, or do you just need to be as good as other people and higher accuracy won’t be a competitive advantage.
What are a few secrets to your success?
Lenses of a polymath: I deeply believe in the value of specialization and putting in the necessary 10.000 hours to become a master in the crafts I decide to pursue. However, the most significant contributions I made in my academic and professional career came from transferring expertise across disciplines: I was the Software engineer that knew more about UX, the ML engineer that knew more about Software, the Quantum researcher that knew more about ML, and the product manager that knew more about Software and deep tech. If you put me head-on against each of these specialists, they may outperform me in their niche. However, my unfair advantages came from the unique combinations that lay in the boundaries across disciplines.
Network is net worth: Although I devour books, papers, podcasts, and beyond, my most revealing insights always come from exchanging with my peers. I always stay present in my ecosystem, let it be by spreading ideas across the internet or taking part in in-person gatherings, conferences, and panels. Moreover, having a mastermind group of peers and mentors with whom I regularly exchange has been one of my biggest learning accelerators.
Love the process: I used to believe in epic goals and conditional happiness. This is like running a car on cheap fuel: It will take you places, but it’s just a matter of time before the engine breaks down. After being close to burning out at the end of my studies, I figured out that a way to sustainable happiness was to love showing up every day. Since then, I have focused more on habits than on targets. Not only do I enjoy my craft more, but I also benefit from a more steady rhythm with results that compound over time.
Who is Raúl Berganza Gómez?
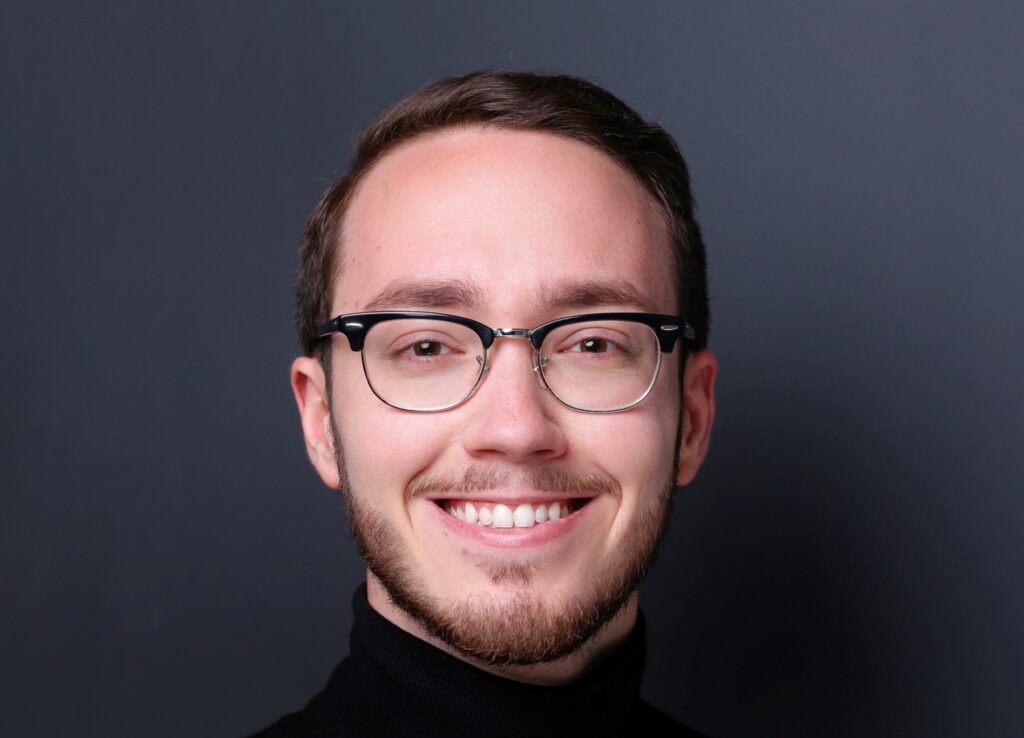
Raúl is a tech-savvy product manager who leads the data governance efforts at the DataOps startup Y42. In his free time, he advocates for tech education as a means of social integration, writes pieces of opinion, and prepares for the next marathon in the closest Berlin park.